Businesses waste tens of thousands of hours per year tracking down the “right” version of their product data and putting it into the “right” format, often duplicating time and effort.
Have you ever planned the perfect meal and then spent too much time searching through a cluttered kitchen for all the ingredients? Disorganized product data presents the same challenges and inefficiencies.
You aren’t looking for any kind of cheese, you are looking for the cheese that you can use to create the perfect meal. Successful PIM implementations and delicious dishes share a common prerequisite: a detailed knowledge of their respective ingredients.
It’s time to standardize the way your team thinks about product data and optimize how you capture your most critical information moving forward. We recommend doing this in three key steps.
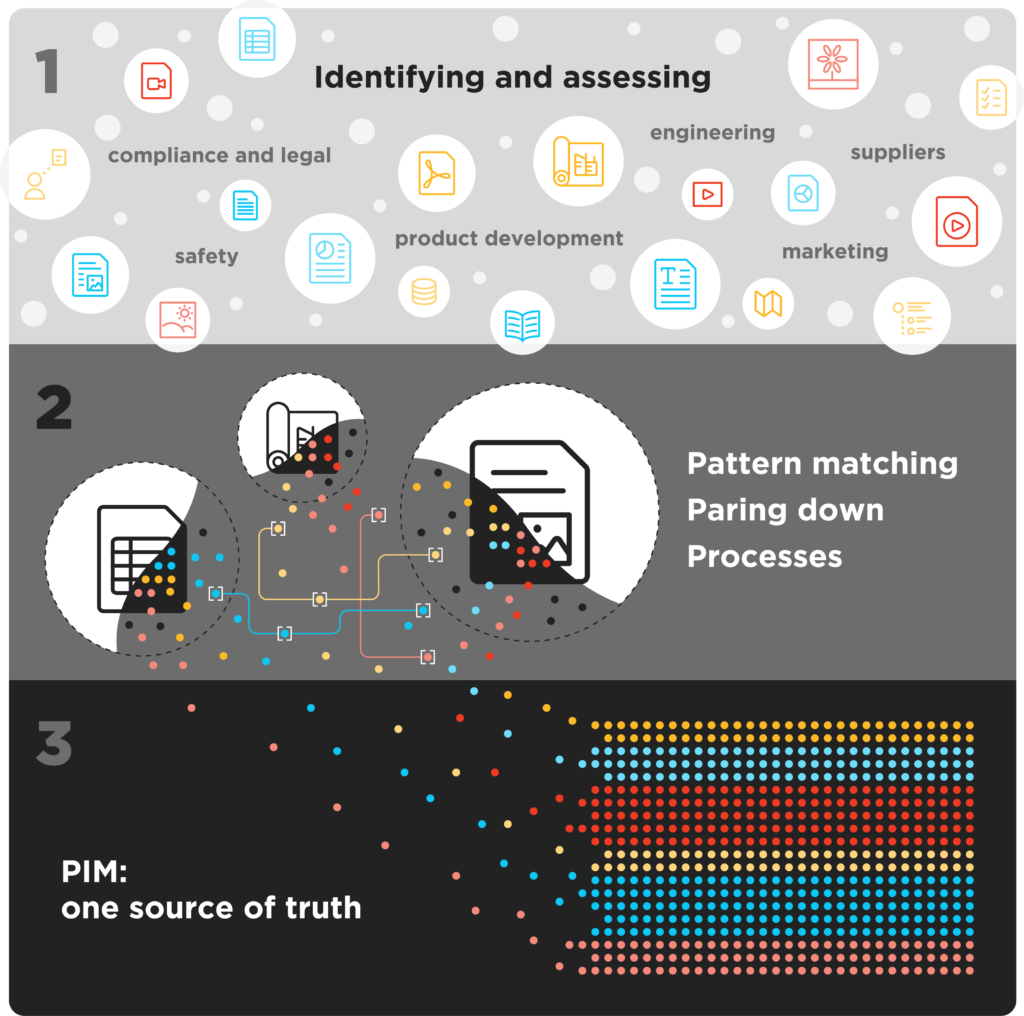
Identifying and assessing product documentation
Before implementing a product information management (PIM) system, product data can exist in multiple places—spreadsheets, PDFs, various internal systems, even inside people’s heads. Countless versions of the same document often exist across the company and within individual departments. And multiple variations of the same data type often exist within each document (e.g., 4.5”, 4.5 inches, 4 1⁄2 inches). The sooner you identify and standardize your product data, the sooner you can begin an efficient PIM implementation.
The first step of product data cleanup is gaining an objective understanding of all the places your data resides today, how organized your data is, and its completeness. Like our kitchen example, the first step to cleanup is to understand all the ingredients that should be in the meal—and sometimes that means checking the back of the fridge.
To accomplish this, we will import your data from all your primary inbound(s), such as an enterprise resource planning (ERP) system, spreadsheets, relational databases, data warehouses, etc. Any data repository can be a potential inbound source.
Once the data has been collected, we analyze it using our established data rubric, scoring it on a scale of 0 to 5 across 5 key data quality categories:
Category |
Description |
Product and item segmentation |
Assesses the ability to place data at the appropriate level to reduce repetition of data elements |
Duplication in data |
Looks for duplication in rows, unique IDs, or unique descriptions |
Data consistency |
Checks to see if data elements, variables, and data types are consistently used to reduce redundancy and maintain clarity |
Data completeness |
Scores the percentage of fields fully populated across all appropriate product and item details to maintain accuracy |
Marketing content |
The availability of content that supports easy marketing, categorization and segmentation of products and items, including product descriptions, features, cross-sells, upsells, parts, kits, bundles, and configurations |
Our rubric examines every column in the dataset, calls out what needs to be fixed, makes recommendations for how to fix it, and asks any remaining questions we have about the data. Then we issue a comprehensive recommendations report that scores each category and averages together each category score to create the overall data cleanliness rating.
In our experience, most businesses think their data is in better shape than it is—but it’s important not to be discouraged by a low score. The good news is that our PIM consultants can advise and guide your team to better data management practices that will produce better data outcomes.
Pattern matching, paring down, and processes
One of the items we examine during the rubric process is pattern matching, which is establishing trends found in the data that can and should be replicated across products and items.
Once a trend has been established, your organization can begin cleansing the data. This includes checking for completeness or deficiencies in product information, removing extraneous legacy data or redundant information, and establishing processes for how to handle data going forward.
Much like only including the freshest ingredients in your perfect meal, we aren’t looking to bring every existing piece of data into the PIM. We only want to bring in the good stuff. For this reason, it’s important to recognize that the patterns discovered and the processes for handling data go hand-in-hand.
For example, “product size” is sometimes managed as an open-text field and pre-PIM your team members entered that information however they wanted. This creates inconsistencies in how “item size” is represented. It might be weight vs. dimensions, or standard vs. metric.
Clean data should be quantitative, standardized, and repeatable. Programmatic conversion of existing open-text data is a good example of leveraging code for standardization. For example, an item’s size was recorded as “2 inches” while another item lists its size as “3 in.” The code can be developed to look for both patterns when cleansing data. After the PIM is implemented, your data experts will have a structured way to enter this data, which decreases the possibility of errors and improves efficiency.
Discovering inefficiencies in the product creation process helps our clients save time and money. For example, if you sell the same pink shirt every spring, it’s best practice to reuse the SKU from the previous year. By eliminating the rework of creating a new SKU for each new year, not only do you save paperwork time, but your data stays cleaner by reducing duplication.
Establishing one source of truth
With your data cleansed and your processes in place, you are now ready to implement your “one source of truth.” While there are several PIM systems to pick from, we are inriver platinum partners. We love inriver because it is highly efficient, it’s extremely customizable, and it can manage outbound expectations from one spot. Inriver can automate many of your current manual processes and allow you to communicate with multiple sales outlets at the click of a button from one system.
Not only does having clean data help facilitate a streamlined PIM project; it’s also critical for your website, ecommerce, communication with the global data synchronization network, and a host of other things. Investing the time up front to cleanse your data will save more than its weight in time and headaches going forward.
Ready to get started?
Poor data quality is the greatest single risk to your PIM project, your ecommerce website, and your downstream channels. It is critical to address the cleanliness of your product data before beginning any PIM implementation or ecommerce project.
To ensure your PIM project goes off without a hitch, you must first assess its cleanliness. At the end of this process, you will have the insight and tools you need for continual clean data management.
To speak with a consultant about your product data, submit the form below. Someone on our team will reach out to schedule a call.